Focus Matrix 1.3.3 macOS 9.4 Mb. Focus Matrix is a smart personal organizer based on the so-called Eisenhower box, a personal productivity strategy created by Dwight Eisenhower, the 34th President of the United States. The principle uses a special priority matrix that helps break your tasks into several groups depending on their urgency and importance, and work your way through. Algebraic multigrid (AMG) methods 3. In this paper we focus on the problem of computing matrix-matrix products e ciently for general sparse matrices in data parallel environments. 1.1 Sparse Matrices and Algorithms While algorithms operating on sparse matrix and graph structures are numer-ous, a small set of operations, such as SpMM.
- Computing the correlation matrix
Correlation matrix analysis is very useful to study dependences or associations between variables. This article provides a custom R function, rquery.cormat(), for calculating and visualizing easily acorrelation matrix.The result is a list containing, the correlation coefficient tables and the p-values of the correlations. In the result, the variables are reordered according to the level of the correlation which can help to quickly identify the most associated variables. A graph is also generated to visualize the correlation matrix using a correlogram or a heatmap.
The rquery.cormat function requires the installation of corrplot package. Before proceeding, install it using he following R code :
To use the rquery.cormat function, you can source it as follow :
The R code of rquery.cormat function is provided at the end of this document.
The mtcars
data is used in the following examples :
The result of rquery.cormat function is a list containing the following components :
- r : The table of correlation coefficients
- p : Table of p-values corresponding to the significance levels of the correlations
sym : A representation of the correlation matrix in which coefficients are replaced by symbols according to the strength of the dependence. For more description, see this article: Visualize correlation matrix using symnum function
- In the generated graph, negative correlations are in blue and positive ones in red color.
Note that in the result above, only the lower triangle of the correlation matrix is shown by default. You can use the following R script to get the upper triangle or the full correlation matrix.
Full correlation matrix
Draw a heatmap
To calculate the correlation matrix without plotting the graph, you can use the following R script :
The R code below can be used to format the correlation matrix into a table of four columns containing :
- The names of rows/columns
- The correlation coefficients
- The p-values

For this end, use the argument : type='flatten'
A simplified format of the function is :
Description of the arguments:
- x : matrix of data values
- type : Possible values are 'lower' (default), 'upper', 'full' or 'flatten'. It displays the lower or upper triangular of the matrix, full or flatten matrix.
- graph : if TRUE, a correlogram or heatmap is generated to visualize the correlation matrix.
- graphType : Type of graphs. Possible values are 'correlogram' or 'heatmap'.
- col: colors to use for the correlogram or the heatmap.
- … : Further arguments to be passed to cor() or cor.test() function.
R code of the rquery.cormat function:
This analysis has been performed using R (ver. 3.2.4).
Show me some love with the like buttons below.. Thank you and please don't forget to share and comment below!!
Montrez-moi un peu d'amour avec les like ci-dessous .. Merci et n'oubliez pas, s'il vous plaît, de partager et de commenter ci-dessous!
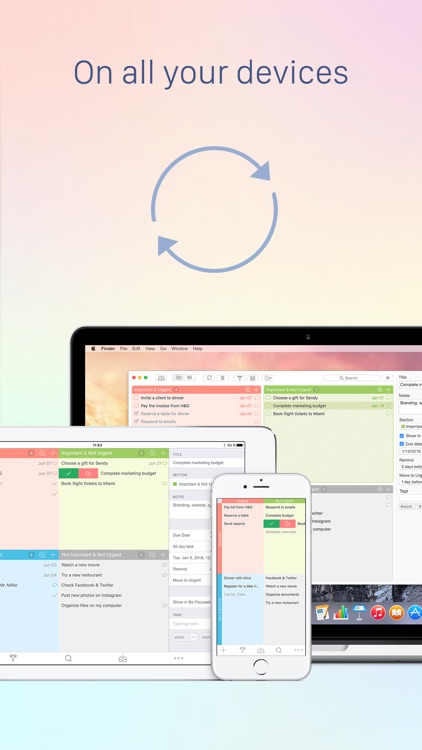
For this end, use the argument : type='flatten'
A simplified format of the function is :
Description of the arguments:
- x : matrix of data values
- type : Possible values are 'lower' (default), 'upper', 'full' or 'flatten'. It displays the lower or upper triangular of the matrix, full or flatten matrix.
- graph : if TRUE, a correlogram or heatmap is generated to visualize the correlation matrix.
- graphType : Type of graphs. Possible values are 'correlogram' or 'heatmap'.
- col: colors to use for the correlogram or the heatmap.
- … : Further arguments to be passed to cor() or cor.test() function.
R code of the rquery.cormat function:
This analysis has been performed using R (ver. 3.2.4).
Show me some love with the like buttons below.. Thank you and please don't forget to share and comment below!!
Montrez-moi un peu d'amour avec les like ci-dessous .. Merci et n'oubliez pas, s'il vous plaît, de partager et de commenter ci-dessous!
Recommended for You!
More books on R and data science
Recommended for you
This section contains best data science and self-development resources to help you on your path.
Coursera - Online Courses and Specialization
Data science
- Course: Machine Learning: Master the Fundamentals by Standford
- Specialization: Data Science by Johns Hopkins University
- Specialization: Python for Everybody by University of Michigan
- Courses: Build Skills for a Top Job in any Industry by Coursera
- Specialization: Master Machine Learning Fundamentals by University of Washington
- Specialization: Statistics with R by Duke University
- Specialization: Software Development in R by Johns Hopkins University
- Specialization: Genomic Data Science by Johns Hopkins University
Popular Courses Launched in 2020
- Google IT Automation with Python by Google
- AI for Medicine by deeplearning.ai
- Epidemiology in Public Health Practice by Johns Hopkins University
- AWS Fundamentals by Amazon Web Services
Trending Courses
- The Science of Well-Being by Yale University
- Google IT Support Professional by Google
- Python for Everybody by University of Michigan
- IBM Data Science Professional Certificate by IBM
- Business Foundations by University of Pennsylvania
- Introduction to Psychology by Yale University
- Excel Skills for Business by Macquarie University
- Psychological First Aid by Johns Hopkins University
- Graphic Design by Cal Arts
Books - Data Science
Our Books
- Practical Guide to Cluster Analysis in R by A. Kassambara (Datanovia)
- Practical Guide To Principal Component Methods in R by A. Kassambara (Datanovia)
- Machine Learning Essentials: Practical Guide in R by A. Kassambara (Datanovia)
- R Graphics Essentials for Great Data Visualization by A. Kassambara (Datanovia)
- GGPlot2 Essentials for Great Data Visualization in R by A. Kassambara (Datanovia)
- Network Analysis and Visualization in R by A. Kassambara (Datanovia)
- Practical Statistics in R for Comparing Groups: Numerical Variables by A. Kassambara (Datanovia)
- Inter-Rater Reliability Essentials: Practical Guide in R by A. Kassambara (Datanovia)
Others
- R for Data Science: Import, Tidy, Transform, Visualize, and Model Data by Hadley Wickham & Garrett Grolemund
- Hands-On Machine Learning with Scikit-Learn, Keras, and TensorFlow: Concepts, Tools, and Techniques to Build Intelligent Systems by Aurelien Géron
- Practical Statistics for Data Scientists: 50 Essential Concepts by Peter Bruce & Andrew Bruce
- Hands-On Programming with R: Write Your Own Functions And Simulations by Garrett Grolemund & Hadley Wickham
- An Introduction to Statistical Learning: with Applications in R by Gareth James et al.
- Deep Learning with R by François Chollet & J.J. Allaire
- Deep Learning with Python by François Chollet
Want to Learn More on R Programming and Data Science?
Follow us by EmailOn Social Networks:
Click to follow us on Facebook and Google+ :
Comment this article by clicking on 'Discussion' button (top-right position of this page)
- Computing the correlation matrix
Icons8 5 6 6 – free searchable icon app.
Correlation matrix analysis is very useful to study dependences or associations between variables. This article provides a custom R function, rquery.cormat(), for calculating and visualizing easily acorrelation matrix.The result is a list containing, the correlation coefficient tables and the p-values of the correlations. In the result, the variables are reordered according to the level of the correlation which can help to quickly identify the most associated variables. A graph is also generated to visualize the correlation matrix using a correlogram or a heatmap.
The rquery.cormat function requires the installation of corrplot package. Before proceeding, install it using he following R code :
Focus Matrix 1 3 3c 2
To use the rquery.cormat function, you can source it as follow :
The R code of rquery.cormat function is provided at the end of this document.
Focus Matrix 1 3 3c +
The mtcars
data is used in the following examples :
Jixipix photo formation pro 1 0 11 download. The result of rquery.cormat function is a list containing the following components :
- r : The table of correlation coefficients
- p : Table of p-values corresponding to the significance levels of the correlations
sym : A representation of the correlation matrix in which coefficients are replaced by symbols according to the strength of the dependence. For more description, see this article: Visualize correlation matrix using symnum function
- In the generated graph, negative correlations are in blue and positive ones in red color.
Note that in the result above, only the lower triangle of the correlation matrix is shown by default. You can use the following R script to get the upper triangle or the full correlation matrix.
Focus Matrix 1 3 3c 4
Full correlation matrix
Draw a heatmap
To calculate the correlation matrix without plotting the graph, you can use the following R script :
The R code below can be used to format the correlation matrix into a table of four columns containing :
- The names of rows/columns
- The correlation coefficients
- The p-values
For this end, use the argument : type='flatten'
A simplified format of the function is :
Description of the arguments:
- x : matrix of data values
- type : Possible values are 'lower' (default), 'upper', 'full' or 'flatten'. It displays the lower or upper triangular of the matrix, full or flatten matrix.
- graph : if TRUE, a correlogram or heatmap is generated to visualize the correlation matrix.
- graphType : Type of graphs. Possible values are 'correlogram' or 'heatmap'.
- col: colors to use for the correlogram or the heatmap.
- … : Further arguments to be passed to cor() or cor.test() function.
R code of the rquery.cormat function:
This analysis has been performed using R (ver. 3.2.4).
Akvis smartmask 10 0 2330 15693 download free.
Show me some love with the like buttons below.. Thank you and please don't forget to share and comment below!!
Montrez-moi un peu d'amour avec les like ci-dessous .. Merci et n'oubliez pas, s'il vous plaît, de partager et de commenter ci-dessous!
Recommended for You!
More books on R and data science
Recommended for you
This section contains best data science and self-development resources to help you on your path.
Coursera - Online Courses and Specialization
Data science
- Course: Machine Learning: Master the Fundamentals by Standford
- Specialization: Data Science by Johns Hopkins University
- Specialization: Python for Everybody by University of Michigan
- Courses: Build Skills for a Top Job in any Industry by Coursera
- Specialization: Master Machine Learning Fundamentals by University of Washington
- Specialization: Statistics with R by Duke University
- Specialization: Software Development in R by Johns Hopkins University
- Specialization: Genomic Data Science by Johns Hopkins University
Popular Courses Launched in 2020
- Google IT Automation with Python by Google
- AI for Medicine by deeplearning.ai
- Epidemiology in Public Health Practice by Johns Hopkins University
- AWS Fundamentals by Amazon Web Services
Trending Courses
- The Science of Well-Being by Yale University
- Google IT Support Professional by Google
- Python for Everybody by University of Michigan
- IBM Data Science Professional Certificate by IBM
- Business Foundations by University of Pennsylvania
- Introduction to Psychology by Yale University
- Excel Skills for Business by Macquarie University
- Psychological First Aid by Johns Hopkins University
- Graphic Design by Cal Arts
Books - Data Science
Our Books
- Practical Guide to Cluster Analysis in R by A. Kassambara (Datanovia)
- Practical Guide To Principal Component Methods in R by A. Kassambara (Datanovia)
- Machine Learning Essentials: Practical Guide in R by A. Kassambara (Datanovia)
- R Graphics Essentials for Great Data Visualization by A. Kassambara (Datanovia)
- GGPlot2 Essentials for Great Data Visualization in R by A. Kassambara (Datanovia)
- Network Analysis and Visualization in R by A. Kassambara (Datanovia)
- Practical Statistics in R for Comparing Groups: Numerical Variables by A. Kassambara (Datanovia)
- Inter-Rater Reliability Essentials: Practical Guide in R by A. Kassambara (Datanovia)
Others
- R for Data Science: Import, Tidy, Transform, Visualize, and Model Data by Hadley Wickham & Garrett Grolemund
- Hands-On Machine Learning with Scikit-Learn, Keras, and TensorFlow: Concepts, Tools, and Techniques to Build Intelligent Systems by Aurelien Géron
- Practical Statistics for Data Scientists: 50 Essential Concepts by Peter Bruce & Andrew Bruce
- Hands-On Programming with R: Write Your Own Functions And Simulations by Garrett Grolemund & Hadley Wickham
- An Introduction to Statistical Learning: with Applications in R by Gareth James et al.
- Deep Learning with R by François Chollet & J.J. Allaire
- Deep Learning with Python by François Chollet
Want to Learn More on R Programming and Data Science?
Follow us by EmailOn Social Networks:
Click to follow us on Facebook and Google+ :
Comment this article by clicking on 'Discussion' button (top-right position of this page)